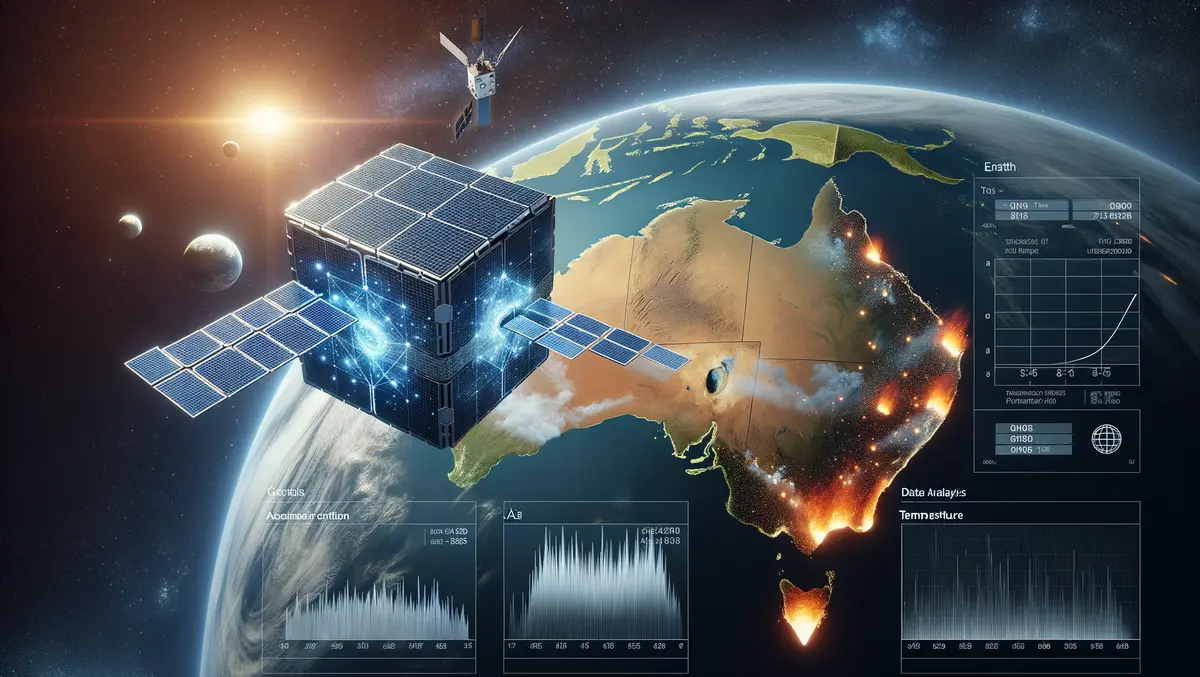
Australian AI cube satellites to revolutionise bushfire detection
Australian scientists are making significant strides in the detection of bushfires, with innovative cube satellites equipped with onboard artificial intelligence (AI) now able to identify fires from space 500 times faster than traditional ground-based imagery processing methods. This development has the potential to revolutionise the response to bushfires, accelerating the detection and allowing quicker ground crew mobilisation, ultimately helping to save lives and properties.
Researchers in remote sensing and computer science have successfully addressed the challenges of processing and compressing large quantities of hyperspectral imagery aboard smaller and more cost-effective cube satellites. By integrating AI, these satellites can analyse the imagery in space before transmitting it to the ground, substantially reducing the time and energy required for data analysis.
This landmark advancement is a result of a project funded by SmartSat CRC and spearheaded by the University of South Australia (UniSA). The initiative has led to the creation of an energy-efficient early fire smoke detection system for South Australia's first cube satellite, named Kanyini. Details of this noteworthy experiment have been published in the latest issue of the IEEE Journal of Selected Topics in Applied Earth and Remote Sensing.
The Kanyini mission, a collaborative effort involving the South Australian government, SmartSat CRC, and other industry partners, aims to launch a 6 U CubeSat satellite into low Earth orbit. Besides bushfire detection, the satellite will also monitor inland and coastal water quality. The onboard hyperspectral imager captures reflected light from Earth across different wavelengths, producing detailed surface maps suitable for diverse applications including land management.
UniSA geospatial scientist Dr Stefan Peters, the lead researcher, highlighted that conventional Earth observation satellites have historically lacked the onboard processing capabilities to analyse complex imagery in real-time. His team, which comprises experts from UniSA, Swinburne University of Technology, and Geoscience Australia, has developed a lightweight AI model capable of detecting smoke within the cube satellite's limited processing, power consumption, and data storage capabilities.
Compared to traditional ground-based processing, the AI onboard model reduced the downlinked data volume to 16% of its original size and consumed 69% less energy. The model also achieved fire smoke detection 500 times faster than the conventional methods. Dr Peters noted the criticality of early detection, observing that smoke is often the first visible indicator from space before the fire intensifies and generates enough heat to be identified by sensors.
The research team utilised simulated satellite imagery of recent Australian bushfires to demonstrate the AI model, training it to distinguish between smoke and clouds efficiently. According to Dr Peters, most sensor systems collect data, only a fraction of which is pertinent to the mission's objectives. Since onboard processing on large satellites is often impractical, all collected data typically needs to be sent to the ground for analysis, a process that is both space-consuming and energy-draining. The newly developed AI model overcomes this by performing essential data processing in space.
A specific case study involved a past fire event in the Coorong region, where the simulated Kanyini AI approach took less than 14 minutes to detect smoke and transmit the data to a ground station at the South Pole. Dr Peters emphasised the substantial benefits of onboard AI compared to traditional ground-based processing, particularly in providing an early warning system for bushfires and other natural disasters.
The researchers plan to demonstrate the onboard AI fire detection system in orbit in 2025, coinciding with the Kanyini mission's operational phase. Future aspirations include commercialising the technology and deploying a constellation of CubeSats to facilitate early fire detection within an hour.
Further insights into the experiment have been documented in the latest issue of the IEEE Journal of Selected Topics in Applied Earth and Remote Sensing.