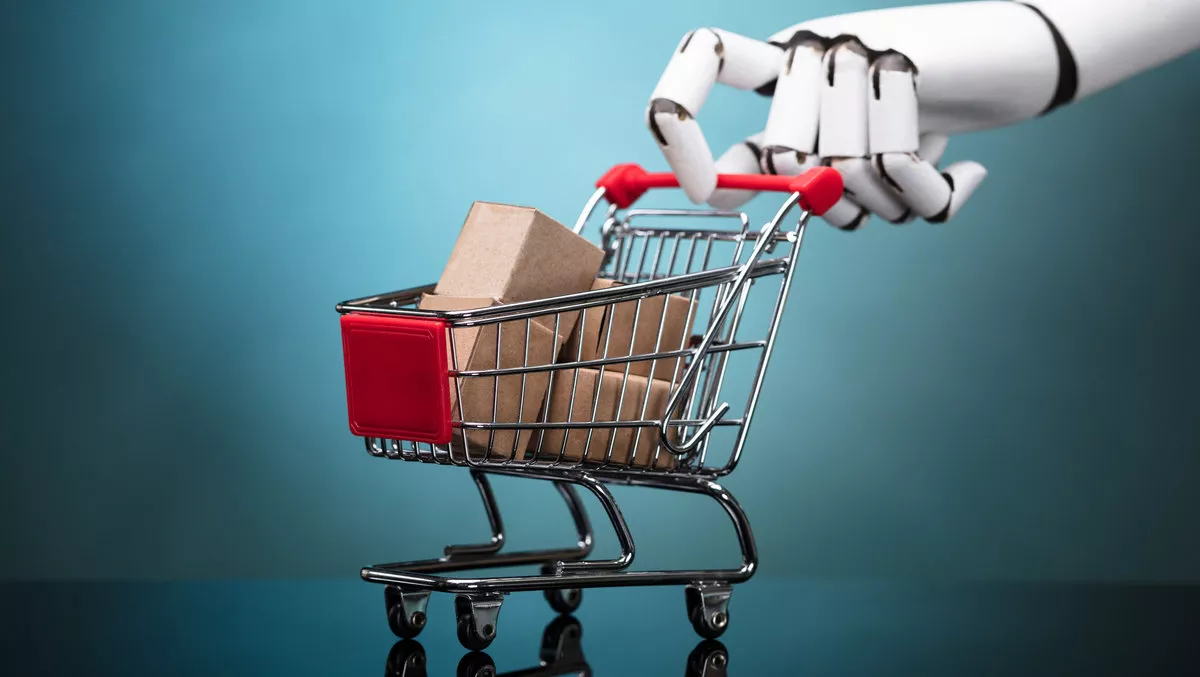
Fujitsu Laboratories has announced the expansion of its "Wide Learning" technology with an innovative AI tool to automatically deliver optimised action plans for users in a variety of fields and industries, including marketing, manufacturing, healthcare, and finance.
Wide Learning machine learning technology forms a core component in Fujitsu Human Centric AI Zinrai, a comprehensive portfolio that encompasses Fujitsu's wide range of AI technologies and techniques.
In the field of marketing, AI has been used to analyse and predict customers behaviour and buying patterns.
While the use of AI to forecast behaviour offers marketers an important way to better understand their customer base, determining actions that encourage purchases remains a challenge.
To address this gap, Fujitsu has expanded its Wide Learning technology, initially developed in September 2018, introducing a new technique that can determine actions that yield a high percentage of purchasers with the minimum number of actions.
The new technique is expected to streamline marketing operations by identifying important customers while simultaneously determining optimal marketing strategies automatically.
In addition to marketing, Fujitsu will also promote this technology in other areas including the automatic control of machines to reduce product defects, automatically setting credit limits to prevent default, and making recommendations for exercise and diet to help users maintain their health.
In recent years, there have been an increasing number of cases in various fields and industries in which the use of AI delivers substantial improvements in operational efficiency.
In the future, it will become possible to further contribute to sales growth and stable production by having AI appropriately propose actions that are highly likely to lead to actual purchases and effective interventions, by applying innovative machine learning techniques to data including anticipated customer segments and conditions of manufacturing equipment, for instance.
When preparing plans for how to approach customers, marketing experts traditionally analyse historical data using segments represented by a combination of data items.
This process typically includes analysis of customer attributes and history of past activity, to estimate actions that may lead to a purchase for a segment with a high purchase rate and a high customer count. However, datasets in marketing often have more than 50 data items, all of which can be combined in quadrillions of potential segment combinations.
In the past, it was only possible to estimate effective sales actions after selecting dozens of segments from the total range of possible combinations.
Going forward, to create more effective marketing strategies, it will be essential to find the most efficient combination of data items that increase the purchase rate for the greatest number of customers with minimal action.
In September 2018, Fujitsu Laboratories developed its Wide Learning AI technology, which can accurately compute high-priority combinations of data items from all combinations even when the amount of data conventionally required for learning cannot be obtained.
Now, Fujitsu has innovated a new technique that further narrows down the large number of calculated high-priority combinations to automatically generate efficient and effective action plans. The features of the new technology are as follows.
1. Identify effective customer segments and actions
By comparing a large number of high-priority combinations of data items that have been calculated with the existing functionality of Wide Learning, combinations are reconstructed to cover many customers and have a high purchase rate.
For example, in marketing, the technique compares several hundred to tens of thousands of segment combinations with high purchase rates to find the combinations that yield the highest possible number of customers without decreasing the purchase rate.
By repeating this process, the user can easily find the few combinations that have the highest headcount and likelihood of purchase, allowing for the rapid identification of the customer segments and appropriate actions needed for an optimal marketing strategy.
2. Choosing actions according to target outcome
When performing an analysis task on combinations of data items, users can assign a priority to each condition, such as "percentage of sample customers that make a purchase," "the number of such persons," or "number of recommended actions".
This makes it possible to choose from actions that meet field targeting objectives such as targeting volume zones and different niches.
Using anonymised data (21 attribute items, 49 action history items, 14 action items) stored in Fujitsu's marketing automation tool (2), an experiment was conducted to recommend actions to increase the number of highly reliable customers.
When Fujitsu's marketing experts analysed the data manually, they found that it was possible to determine 14 segments with actions that cover 17% of purchased customers and have 55% purchase rate on average.
By applying the new technique to the same data it became possible to determine 3 segments with actions that cover 47% of customers with an average 92% purchase rate.
By the end of March 2020, this technology will be used to automatically determine the best way to deliver information on products and services to customers, as well as to provide information on events hosted by Fujitsu.
In 2020, Fujitsu also aims to use the technology in its "FUJITSU Intelligent Data Service AD Drive Operational Marketing Service" which delivers advertisements over the Internet. Aside from marketing, Fujitsu will analyse the relationship between business segments and actions in fields including manufacturing, finance, healthcare, and more in order to improve operations through optimising action plans.
Fujitsu has added this technique into its Wide Learning offering and is currently integrating it into various Fujitsu products and services with the aim of providing it as a Zinrai solution by fiscal 2020.