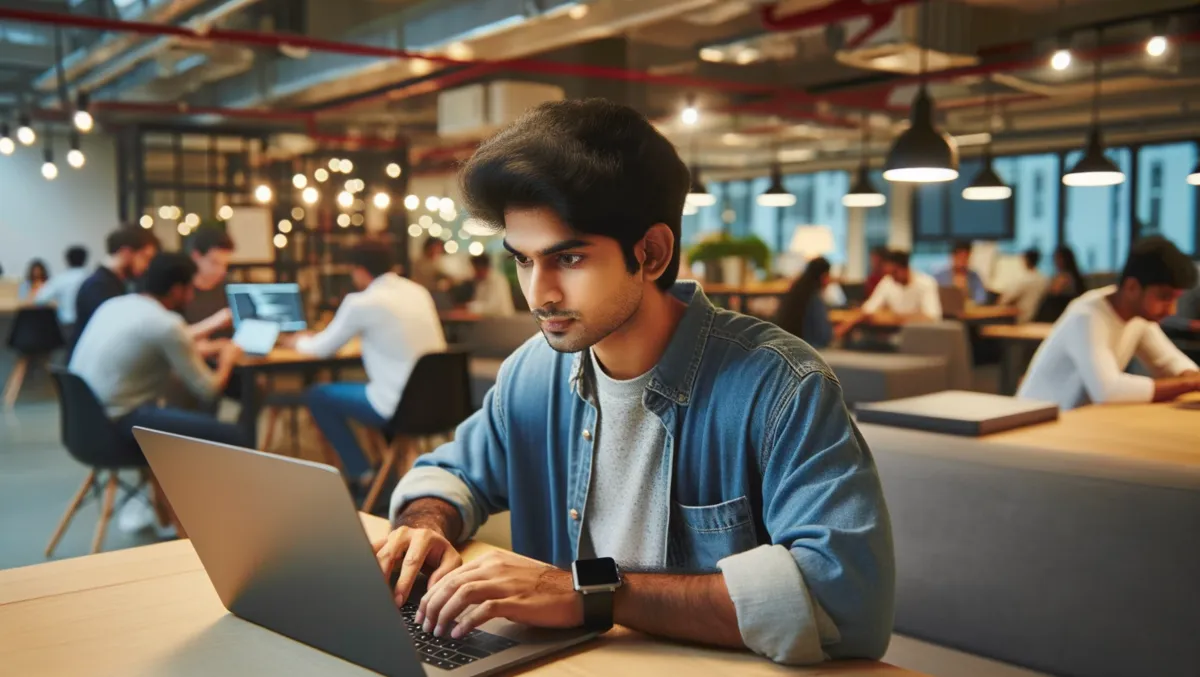
DataStax unveils RAGStack to simplify generative AI application development
DataStax, a company that powers generative AI applications with real-time, scalable data, has announced the launch of RAGStack, a new, innovative retrieval augmented generation (RAG) solution. The product launch has been met with anticipation as it seeks to simplify the implementation of RAG applications designed with LangChain.
RAG applications provide context from external data sources to generate accurate responses from large language models (LLMs). However, their implementation can prove overwhelming due to the complex selection of tools and frameworks available. Demonstrating its understanding of the struggles faced by developers, DataStax's introduction of RAGStack is designed to reduce complexity, offering a streamlined, tested, and efficient set of tools and techniques for building with LLMs.
RAGStack positions itself as a preselected and ready-made solution for developers, using the best open-source software to implement generative AI applications. The solution uses the LangChain ecosystem, which includes LangServe, LangChain Templates, and LangSmith, along with Apache Cassandra and the DataStax Astra DB vector database. The implementation of these popular tools aims to remove the hurdles of bespoke solution assembly and offers developers a simplified, comprehensive generative AI stack.
Harrison Chase, CEO of LangChain, commended DataStax for addressing a critical pain point in the market: "Every company building with generative AI right now is looking for answers about the most effective way to implement RAG within their applications. DataStax has recognised this issue and is working to remedy the problem with the release of RAGStack. Using top-choice technologies, like LangChain and Astra DB among others, DataStax is providing developers with a tested, reliable solution made to simplify working with LLMs."
RAGStack blends the strengths of both retrieval-based and generative AI methods to generate contextually relevant responses in real time. These advancements greatly influence the innovative leaps taking place in this technology today. RAGStack enhances existing vector search techniques and is compatible with most generative AI data components, which overall improve the performance, scalability, and cost of implementing RAG in generative AI applications.
Sandeep Penmetsa, head of data science and engineering at PhysicsWallah, highlighted their utilisation of RAGStack in their work: "At PhysicsWallah, we're dedicated to delivering high-quality and affordable education. We built a generative AI-driven chatbot powered by the Astra DB vector database and LangChain to be a one-stop solution for every student's learning needs. We employ Astra DB’s semantic search for advanced support queries, enriching our students' learning experience, and RAGStack facilitates seamless deployment of RAG-based applications."
Similarly, Tisson Mathew, CEO of Skypoint, highlighted the potential benefits of RAGStack for their healthcare AI solution: "DataStax technology is deeply integrated into our generative AI infrastructure. We've built our solution with Astra DB and customised open source software like LangChain – this is what we have in production today. With RAGStack, we'll be able to reduce the pain of maintaining customised open source software, helping to deliver a more simplified and streamlined healthcare AI solution for our customers."
Davor Bonaci, CTO and executive vice president at DataStax, also emphasised the importance of RAGStack in the currently congested marketplace: "Out of the box RAG solutions are in high demand because implementing RAG can be complex and overwhelming due to the multitude of choices in orchestration frameworks, vector databases, and LLMs. It's a crowded arena with few trusted, field-proven options, where demand is high, but supply is relatively low. RAGStack helps to solve this problem and marks a significant step forward in our commitment to providing advanced, user-friendly AI solutions to our customers."